Online Master of Science in Computer Science Program
Request Information
Online MS in Computer Science Program Overview

20-36 Months To Complete*
*Varies based on class availability and the number of credit hours taken per semester.

$2,027 per Credit Hour**
Understand tuition and financial aid
**Based on 2024-2025 tuition rate. Tuition and fees subject to change each year as approved by Tulane University
How long does it take to complete the Online Master of Science in Computer Science?
The time it takes to earn your MSCS online from Tulane depends on your program pace and individual priorities. You have the option to complete the 10-course, 30-credit hour MSCS at a full- or part-time pace. A full-time pace requires taking two to three courses per semester for five semesters. In this case, you can complete your degree in an average of 20–28 months.
Alternatively, a part-time pace is a manageable workload if you plan to continue working while earning your degree. A recommended part-time pace is one to two courses per semester, which would allow you to complete your degree in approximately 28–36 months. For full- and part-time learners, your weekly time commitment consists of live classes, independent coursework, and study time.
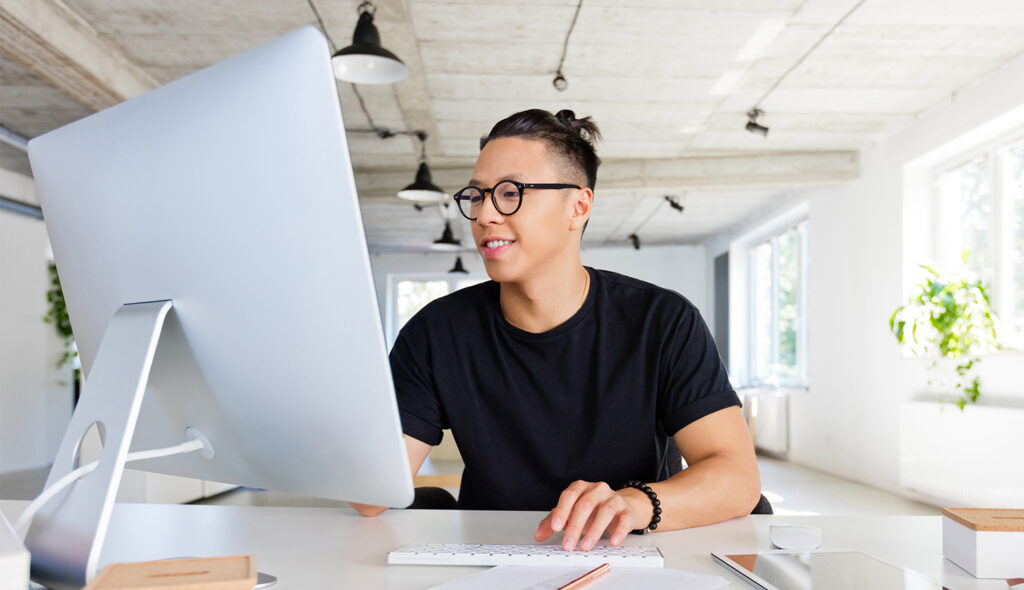
Is this program the right fit for you?
At Tulane, our online master’s in computer science program offers you the option to customize your coursework and areas of emphasis. So whether you’re a seasoned computer scientist or an aspiring artificial intelligence specialist, our degree has something for you.
Prepare for your professional pivot
For technical professionals interested in changing industries, the online master’s in computer science can equip you with the tools to pivot. If you have a STEM bachelor’s degree and are curious about computer science, our Online MSCS program will prepare you with the depth of technical know-how and industry connections you need to succeed in your next role.
Advance in your industry
Today, an Online MS in Computer Science degree is a typical requirement for computer research scientist roles.* If you’re already in the field and eager to advance, our online computer science master’s can help you reach your goals. Develop next-level computing skills, with the option to focus your studies on natural language processing, machine learning, algorithms, and more. Review our career outlook page for a more comprehensive look at the career paths available to you upon graduation.
*U.S. Bureau of Labor Statistics, 2022
Find your specialty
Sights set on a career in research or academia? The computer science master’s degree faculty will help you prepare for your dream job. Our graduate degree also offers you the option to dive deeper into areas like computational geometry, cloud computing, data science, and beyond so that you can become a best-in-class specialist upon graduation.
Online Program Experience
Tulane’s Online Master of Science in Computer Science program redefines flexibility. Here, it means you can complete your online master’s in computer science from wherever you are, at your own pace, all while choosing electives connected to your interest areas.
You are at the center of our Online MS in Computer Science program. As an online student, you will participate in virtual classes with professors and peers while you complete self-paced, project-based assignments on your own schedule. Our small class sizes mean you’ll work closely with your faculty and classmates throughout the program. You can ask questions, share insights, and receive feedback, just like you would taking classes on campus. There are also live office hours to further your connection to faculty and support your mastery of program concepts.
The typical weekly time commitment for the Online MSCS varies based on the number of credit hours taken each semester. On average, you can expect to spend two to three hours per week, per credit hour, on homework and assignments. This includes one 75-minute live session per three-credit-hour course each week.
For more information about the online structure and program experience, schedule time with one of our enrollment advisors today.
Sample Curriculum
What will you learn in a computer science master’s program?
Tulane’s Online MS in Computer Science curriculum challenges you to think beyond basic programming. With an increase in remote work and demand for virtual operational systems, today’s computer science professionals need a wide variety of skills.
Our 30-credit curriculum prepares you with the tools you need to make the most of the expanding digital space while focusing on areas of the field that interest you. Over the course of your degree program, you will complete nine credits of core classes and 21 elective credits based on your chosen focus area.
Focus areas include:
- COMPUTATIONAL GEOMETRY
- COMPUTATIONAL BIOLOGY AND INFORMATICS
- ALGORITHMS AND THEORY
- SYSTEMS
- DATA SCIENCE
- ARTIFICIAL INTELLIGENCE AND MACHINE LEARNING
Please note that Online MSCS courses are subject to change and not every course will be offered every semester.
Bridge Course
This class focuses on several core topics in the design, analysis, and implementation of computational tools that are drawn from the fields of data structures, software engineering, and programming languages. Other topics include object-oriented programming, test-driven development, data structures and abstract data types, imperative programming and memory management, and functional programming.
By solving practical, real-life problems in different programming languages and in different ways, students learn to select a language and approach most appropriate for the situation, and prepare to learn new languages independently. The high-level goal of this course is to train students to be able to draw from a versatile set of skills, which in turn will provide a strong foundation for further study in computer science.
Core Courses
This course covers fundamental algorithm design principles and data structures, basic notions of complexity theory, as well as an advanced introduction to parallel algorithms, randomized algorithms, and approximation algorithms. Topics include: divide-and-conquer, dynamic programming, amortized analysis, graph algorithms, network flow, map reduce, and more advanced topics in approximation algorithms and randomized algorithms. Satisfies the algorithm breadth area requirement.
This course is designed for graduate students interested in understanding the design of autonomous intelligent agents. The course will cover fundamental notions and concepts such as uninformed and informed search, local search, constraint satisfaction and constraint-based optimization, Bayesian Networks, Markov Decision Problems and a short introduction on machine learning. Furthermore, advance topics and applications in the context of natural language processing, reasoning about time, algorithmic game theory and computational social choice will be considered as well.
This course covers the design and implementation of operating systems. Operating systems serve as an interface between the hardware of a computer and the software running on it. This course addresses how operating systems enable the sharing of limited resources — CPU, memory, hard disks — robustly, securely, and efficiently amongst all running processes on a computer. CPU virtualization, concurrency, memory virtualization, file systems APIs and implementations, and security are all covered with a focus on the key design ideas and abstractions within the topics. In addition, students get practical experience implementing their own operating system.
The objective of the course is to introduce students to the core concepts and analytic techniques in the design and analysis of computer networks and network protocols. We will explain both how computer networks work using the Internet as the paradigm and why they work from an optimization and control perspective.
Elective Courses
This course provides an introduction to geometric algorithms and geometric data structures. Computational Geometry is a young discipline which enjoys close relations to mathematics and to various application areas such as geometric databases, molecular biology, sensor networks, visualization, geographic information systems (GIS), VLSI, robotics, computer graphics and geometric modeling. Covered topics include fundamental geometric algorithm design and analysis paradigms, geometric data structures for planar subdivisions and range searching, algorithms to compute the convex hull, Voronoi diagrams, and Delaunay triangulation, as well as selected advanced topics.
This is a hands-on introductory security course for upper-level undergraduate students and graduate students. Students will learn the basics of cryptography and methods for protecting systems from attack. We will cover malicious software and other attacks that occur over the network, as well as the perimeter defenses used to stop these attacks. Students will then learn about program vulnerabilities that lead to most of the security problems in computing today. We will conclude with the other administrative issues that security professionals must consider in their jobs.
This course is an introductory course on the fundamentals of 3D game development. We will focus on the Unity game engine and object-oriented programming with C#. Students will learn various topics including vectors and rotations, character animation, artificial intelligence, and Unity tools, such as Cinemachine, Shader Graph, and Timeline.
This course is designed for both graduate students and advanced undergraduate students interested in understanding of both the fundamental and advanced concepts, techniques, and technologies required for collecting, processing, and deriving insight into data. Data Science is an interdisciplinary set of topics that includes everything you need to create data driven answers and solutions to specific business, scientific, or sociological questions. Topics typically covered include an introduction to one or more data collection and management systems, e.g., SQL, web scraping, and various data repositories; exploratory and statistical data analysis, e.g., bootstrapping, measures of central tendency, hypothesis testing and machine learning techniques including linear regression and clustering; data and information visualization, e.g., plotting and interactive charts using various technologies; and presentation and communication of the results of these analyses. Students should be comfortable programming in Python and familiar with the fundamentals of algorithmic analysis and computer systems.
A comprehensive introduction to the mathematics and algorithms that drive today’s digital special effects, animation, and games. Designed as a hands-on course, students will gain experience in building 2D/3D interactive applications using OpenGL. Topics covered will include geometric transformations, projections, raster algorithms, 3D object models (surface and volume), visible surface algorithms, texture mapping, lighting/shading, ray-tracing, anti-aliasing, and compositing.
This course investigates computational methods to work with human language, analyzing its lexical, syntactic, and semantic aspects. Examples include document classification and clustering, syntactic parsing, information extraction, speech recognition, and machine translation. Theoretical and practical aspects of the latest techniques will be covered, including probabilistic modeling, neural networks, and deep learning.
Structure and organization of computer systems; instruction sets; arithmetic; data path and control design; memory hierarchy.
Faculty Spotlight
Meet some of the brilliant minds behind our Online MS in Computer Science at Tulane. Internationally recognized researchers and practitioners, the MSCS faculty bring a high caliber of computer science proficiency to the classroom.